Understanding Statistics: A Comprehensive Guide to Concepts and Applications
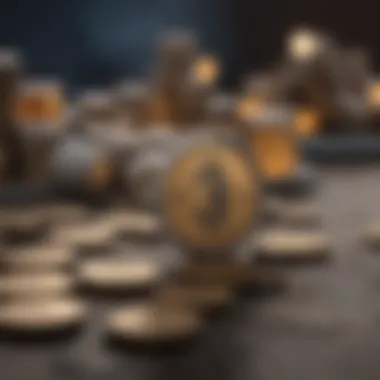
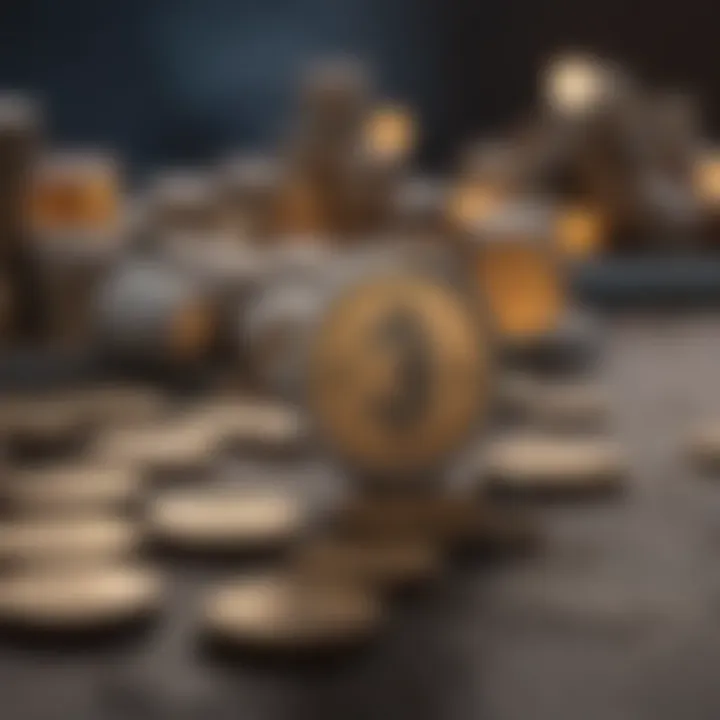
Intro
Statistics is a field often overlooked, yet it permeates our daily lives more than one might think. From interpreting the polls we read, to understanding the data behind scientific experiments, statistics guides decision-making across various sectors. It provides a backbone to many disciplines, including healthcare, social sciences, business, and sports.
Beginning this journey through the world of statistics, itās essential to singal out the fundamental terms and their implications. Securing a grasp of the key terminologies lays a vital foundation that enables deeper insight into broader aspects of the field.
Key Terms and Definitions
When diving into the world of statistics, familiarizing oneself with essential terms can be like finding your footing on a rocky path. Here are some key terms that serve as the cornerstones of the statistical lexicon:
- Population: In statisitcs, this refers to the entire group that you want to draw conclusions about. You can think of it as the full cast in a theatrical play, embodying every character.
- Sample: A sample is a subset of the population, collected for observation and analysis. Itās akin to choosing a few actors for a scene, rather than watching the whole performance.
- Mean: Commonly known as the average; you compute it by adding all values and dividing by the total number of values. Picture it as a middle point among a group of numbers.
- Median: This is the middle value when numbers are arranged in order. If you had a line of people sorted by height, the median would be the one standing right in the middle.
- Mode: The mode indicates the most frequently occurring value in your data set. If you had a jar full of marbles of varied colors, the mode would be the color with the most marbles.
- Variance: Variance measures the extent to which each data point differs from the mean. Itās like looking at how all the actors in your play stray from the usual character traits theyāre expected to show.
- Standard Deviation: This is the square root of variance, and it gives an idea of how spread out the values are in your data set, much like mapping out the distances from the stage in a theater to where the audience is sitting.
Explanation of Investment Terminology
In the financial realm, statistical terminology takes on specialized forms, often critical for investors.
- Return on Investment (ROI): A measure of the profitability of an investment. It tells you how much you gain or lose compared to what you put in.
- Diversification: In finance, this refers to spreading investments across various financial products to mitigate risk. Itās like not putting all your eggs in one basket.
- Volatility: This term refers to the degree of variation in a trading price series over time. High volatility means big price changes and risks, while low volatility indicates relatively stable prices.
By understanding these basic terms, you start gaining the upper hand in navigating through statistical data and financial discussions.
head> title>Statisticstitle> link rel= "stylesheet" href= "https://www.stat.berkeley.edu/" type= "text/css" head>
From grasping fundamental terms to understanding the complexities of variances and standard deviations, each step you take in learning statistics opens doors to wiser, more informed decisions.
Ending
Arming oneself with statistical knowledge not only enhances decision-making capabilities but also fosters a mindset capable of analyzing trends and patterns hidden in masses of data. Remember, it is key to distance oneself from simple interpretations and appreciate the rich, nuanced information that statistics offers.
Foundations of Statistics
The foundations of statistics act as the bedrock for understanding data and making informed decisions. In any field of study, be it health, finance, or social sciences, having a grasp on statistical principles is crucial. Statistics is not merely about calculating numbers; it is about interpreting those numbers to gain insights. This section aims to dissect the elemental concepts that define statistics and highlight why they hold significance in practical scenarios.
Definition and Purpose of Statistics
Statistics can be defined as the branch of mathematics that deals with data collection, analysis, interpretation, presentation, and organization. Its primary purpose is to summarize complex data sets into manageable information that can be easily understood and communicated. For instance, when a researcher collects data on patient outcomes, they rely on statistical methods to derive conclusions that inform medical practices. Without statistics, raw data would be like an unsolved puzzle, offering no coherent picture of the underlying trends.
In practice, the definitions of statistical terms, like 'mean', 'median,' and 'variance', serve as key components. Hereās a quick rundown of these essentials:
- Mean: The average of a set of numbers, found by dividing the sum by the count.
- Median: The middle value when a data set is arranged in order.
- Variance: Measures how far a set of numbers is spread out from their average value.
Each term possesses its purpose and utility, helping to draw nuanced conclusions from the data available.
The Importance of Statistics in Everyday Life
Statistics permeates daily existence in subtle ways, influencing decisions made at every level of society. From the advertisements we see in our social media feeds to government policies shaping public health, the application of statistics is both vast and vital. Here are some examples that underscore its importance:
- Health and Medicine: Clinical trials for new medications rely heavily on statistical methods to determine effectiveness and safety. Without these analyses, new treatments would lack the necessary backing.
- Economics: Government entities use statistics to analyze economic data and forecast future trends. This forecasting directly impacts job creation, taxation policies, and consumer rights.
- Sports: Team managers use statistics to make decisions about player trades, game strategies, and performance evaluations. Just think how each playerās stats can influence the choices made by a team.
"In today's data-driven world, the ability to understand and apply statistics can significantly enhance decision-making across multiple domains."
In sum, the relevance of statistics in everyday life cannot be overstated. It equips individuals with the tools to interpret data critically, fostering an informed society capable of making reasoned decisions.
Types of Statistics
In the realm of data analysis, understanding the types of statistics is critical. Statistics can be broadly categorized into two main types: descriptive statistics and inferential statistics. Each type serves a unique purpose and offers distinct methods for analyzing and interpreting data, which is essential for drawing meaningful conclusions in various fields. Understanding these types equips individuals with valuable tools for not just processing numbers, but also for making informed decisions based on empirical evidence.
Descriptive Statistics
Descriptive statistics provide a summary of the data at hand. This summary can take several forms, primarily through measures of central tendency and measures of dispersion.
Measures of Central Tendency
Measures of central tendency are a way to describe the center point of a dataset. This includes means, medians, and modes. The mean offers a simple average, but it can be skewed by outliers. Conversely, the median represents the middle value and is often viewed as a more robust measure when there are extreme values at play.
The appeal of using measures of central tendency lies in their simplicity. Stakeholders can quickly grasp the key characteristics of the dataset at a glance. However, a unique downside is that these measures alone do not provide a comprehensive view of the data; they're just the tip of the iceberg. For instance, knowing the average income in a neighborhood isn't enough to understand economic disparity if that average is largely influenced by a handful of very high earners.
Measures of Dispersion
Measures of dispersion, on the other hand, offer insights into the distribution of data points and variability. Common measures include the range, variance, and standard deviation. Understanding dispersion is crucial as it informs how spread out the data points are; a high standard deviation signals that values are widely dispersed from the mean, which could indicate underlying complexity in the dataset.
This specific aspect makes measures of dispersion indispensable in this narrative. They complement measures of central tendency, providing a fuller picture of the data landscape. A dataset could have the same mean but vastly different standard deviations, leading to diverse interpretations.
Inferential Statistics
Moving beyond mere description, inferential statistics calculate probabilities to make predictions about a larger population from a sample. This section includes crucial elements such as sampling methods and estimation and hypothesis testing.
Sampling Methods
Sampling methods dictate how data is collected for analysis. Techniques such as random sampling or stratified sampling play a pivotal role in ensuring that the sample reflects the population accurately. This is worth emphasizing, as the integrity of the sampling method can heavily influence the reliability of the results.
A notable characteristic of good sampling methods is that they allow for broader inferences without the need for every individual in the population to be tested. However, the unique feature here lies in the potential biases that can arise from poor sampling techniques. If a sample is not representative, the conclusions drawn can be misleading, which is a significant drawback worth considering.
Estimation and Hypothesis Testing
Estimation and hypothesis testing are at the heart of inferential statistics. They involve making predictions about the population parameters based on sample data. This practice enables statisticians to draw conclusions while accounting for uncertainty, which is a frequent occurrence in data analysis.
The concept of hypothesis testing revolves around formulating a null hypothesis and an alternative hypothesis, and determining which one is more plausible based on the data. However, this method is not without challenges. One major advantage is that it allows for quantifiable testing of assumptions, but it also carries the risk of Type I and Type II errorsārejecting a true null hypothesis or failing to reject a false null hypothesis, respectively. This highlights the thought necessary when interpreting results and their implications.
Data Collection and Analysis
Data collection and analysis are the backbone of statistical practice. This stage is where raw information transitions into valuable insights. Quality data lays the groundwork for any analytical work. If you donāt collect the right data, it wonāt matter how good your analysis is. The benefits of solid data collection cannot be overstated. It not only refines the findings but ensures they are credible and actionable. When collecting data, a variety of elements come into play, including the purpose of the data, the methods of collection, and the context in which the data will be analyzed.
Designing Effective Surveys
Crafting an effective survey isn't just about shooting questions out into the ether. It's a careful art that merges science and intuition. A well-designed survey can elicit responses that are insightful and well-rounded. This process begins with defining clear objectives. What exactly do you want to discover? Without a focused aim, itās like shooting in the dark.
Once you have clarity on your objectives, the next step revolves around crafting questions that are concise and unbiased. Here are a few tips to keep in mind:
- Keep it simple: Avoid jargon. Questions should be clear and accessible.
- Be specific: Ambiguity leads to mixed responses. Precisely pin down what you are asking.
- Question types: Use a mix of open-ended and close-ended questions to gather both quantitative and qualitative data.
In addition, piloting your survey can reveal unanticipated issues. A small trial run helps in identifying potential pitfalls that might confuse respondents or skew your data.
Experimentation and Observational Studies
When it comes to examining phenomena, experimentation and observational studies serve two distinct yet equally critical roles. Experiments allow researchers to manipulate one variable to see how it affects another. This cause-and-effect approach is powerful, providing clarity on relationships among variables. For instance, in a medical trial, one group might receive a new medication while another group does not. The outcomes can illuminate the medication's efficacy.
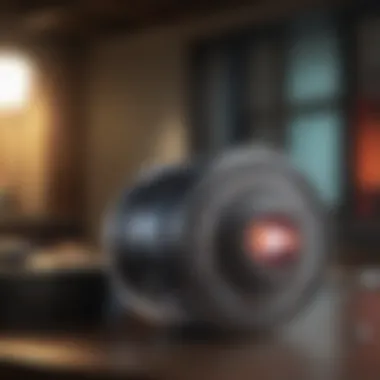
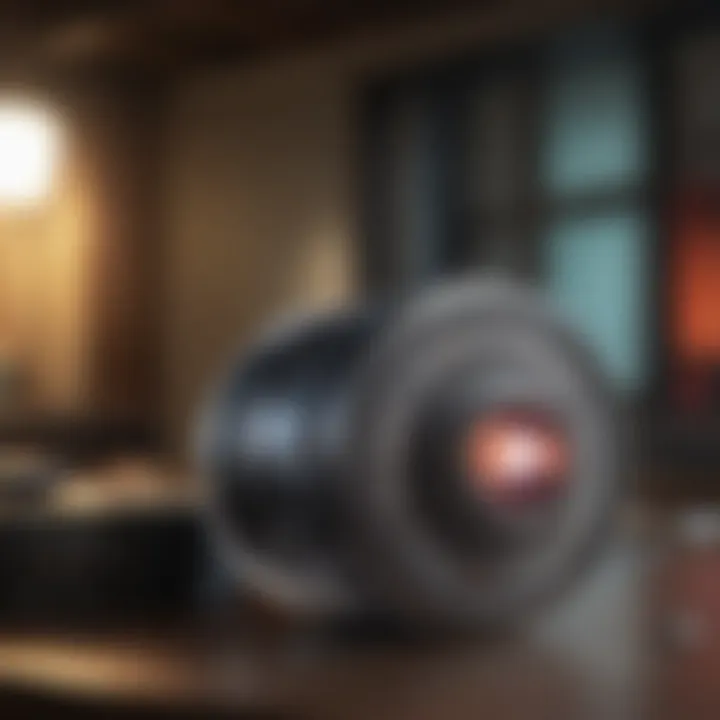
On the flip side, observational studies can reveal trends and correlations without diving into the world of manipulation. They provide insight into real-world situations as they unfold naturally. This could involve surveying peopleās lifestyles and health outcomes over time. These studies are invaluable when ethical considerations restrict experimentation.
Data Cleaning and Preparation
Data cleaning is akin to polishing a rough stone into a glittering gem. Itās a step that is often overlooked but is crucial in ensuring the integrity and accuracy of data analysis. No one wants to base conclusions on flawed data. Common issues include irrelevant responses, duplicate entries, and missing values. Thus, data cleaning involves scrutinizing the data set and making it fit for analysis.
Here are some steps for effective data cleaning:
- Remove duplicates: These can skew your results and misrepresent phenomena.
- Handle missing values: Decide whether to fill in gaps, remove the affected entries, or leave them as is based on analysis goals.
- Standardize formats: Ensure consistency across the data set. For instance, having dates in a single format can save a lot of headaches later.
As a result of thorough data cleaning, researchers can present findings with greater confidence. Itās in this stage where messy and unorganized data transforms into clean, analyzable, and meaningful information.
"Clean data is not just a nicety; it's an absolute necessity for sound statistical analysis."
Data collection and analysis form a vital cycle. You design, collect, clean, and finally analyze, leading to informed decisions based on actionable insights. Understanding these processes enhances your ability to leverage statistical methods effectively, whether you're a novice or seasoned in the field.
Probability Concepts
Probability is the backbone of statistics, playing a critical role in helping us make sense of uncertainty in data. Understanding how likelihood and chance operate equips individuals with tools to decode complex scenarios, whether in business, healthcare, sports, or daily life. It shapes decision-making processes and allows for more informed conclusions derived from data. Grasping probability concepts lays the groundwork for statistical reasoning, making it an essential topic in this guide.
Understanding Probability
Basic Probability Rules
Basic probability rules govern the likelihood of occurrences in a given context. They guide reasoning by providing a structured way to understand how likely events are to happen. The central tenet of these rules is that probabilities range between 0 and 1, where 0 signifies impossibility and 1 indicates certainty. This characteristic makes it intuitive for beginners as it serves as an entry point to the world of statistics.
One of the hallmark elements of these rules is the Addition Rule, which states that the probability of either A or B happening is the sum of the probabilities of A and B, minus the probability of both occurring. For example, when rolling a dice, the chance of rolling either a 2 or a 3 combines the individual probabilities of 2 and 3. This kind of clarity makes it a popular choice for anyone starting in statistics.
The nuance lies in using these rules properly; misapplying them can lead to errors in judgment. As such, understanding their unique features is vital. The main advantage of adhering to basic probability rules is their simplicity, but a disadvantage is that they can become complex in multi-event scenarios. Therefore, a solid grasp of these foundational concepts is key.
Conditional Probability
Conditional probability takes a step further by considering the probability of an event given that another event has occurred. This concept is particularly significant in situations where dependencies existālike in medical testing or risk assessments. For instance, if you want to determine the probability of a disease after a positive test result, the context is critical.
The key characteristic of conditional probability is its reliance on prior information. This makes it a valuable tool for understanding real-world phenomena, indicating itās a beneficial framework for analysis in this article. Its unique feature lies in how it uses existing knowledge to refine probability assessments. A useful example might be that if one knows that a certain demographic is more prone to a disease, their chance of finding a positive result increases.
However, with this power comes a responsibility: misinterpreting or failing to consider all variables can lead to skewed perceptions. Thus, while conditional probability serves as a critical piece of statistical analysis, it requires careful consideration of context when making sense of results.
Probability Distributions
Probability distributions are fundamental constructs in statistics, providing a model to describe how probabilities are spread over outcomes. These distributions tell us what to expect in terms of data behavior, helping in effective forecasting and decision-making.
Normal Distribution
The normal distribution, often referred to as the bell curve, is a key feature of statistics. Its significance arises from the central limit theorem, which states that, given enough trials, the sample mean will be normally distributed regardless of the original distribution of the population. This characteristic makes it a popular topic in statistics because it facilitates various analyses.
One unique feature of the normal distribution is its symmetry around the mean. This property allows statisticians to make inferences and derive conclusions about population parameters from sample statistics with relative ease. While it has notable advantages, such as simplicity and applicability, reliance on normal distribution also has disadvantages, particularly when dealing with skewed data or outliers that can distort results.
Binomial Distribution
The binomial distribution models a scenario with two possible outcomes, typically labeled as success and failure. Its importance lies in its application in a variety of fields; for instance, in predicting the outcomes of tests or elections where only two results are plausible.
A core characteristic is its definition in terms of 'n' trials and the probability of success 'p'. It is beneficial to understand this aspect because it neatly aligns with real-life scenarios, such as flipping a coin. However, while the binomial distribution offers clarity, it assumes equality of outcome probability throughout its trials, which can sometimes oversimplify complex situations.
Ultimately, mastering these distributions contributes significantly to the overall understanding of statistics and aids in applied analysis.
Statistical Inference
Statistical inference serves as a bedrock for understanding how to make predictions and draw conclusions about a population based on sample data. This technique is vital because it enables statisticians and researchers to go beyond mere description of collected data. In essence, it allows us to generalize findings from a sample to a larger population, which is incredibly powerful in various fields, including business, healthcare, and social sciences. By leveraging statistical inference, one can derive insights, test assumptions, and make decisions that are informed rather than arbitrary.
Here, we will delve into key elements such as confidence intervals and the hypothesis testing framework, both vital tools in the statisticianās arsenal.
Confidence Intervals
A confidence interval offers an estimation range that is likely to contain the population parameter. This range derives from a sample statistic, providing an adjustable level of certainty ā usually expressed as a percentage. For instance, an analyst might say they are 95% confident that the true population mean lies between two specific values. Itās akin to casting a net in a sea of uncertainty; while you may not know the exact catch, you have a good sense of where to look.
Having a confidence interval is crucial as it not only reflects the precision of the sample estimate but also helps in decision-making processes. If a business wants to understand consumer preferences, rather than rely solely on a single point estimate, examining the confidence interval gives a clearer picture of where opinions may range.
Hypothesis Testing Framework
Hypothesis testing operates as the glue that binds numbers to conclusions. This framework allows one to test assumptions about a population based on sample data. At the heart of this process are two conjectures: the null hypothesis, which posits that no effect or no difference exists, and the alternative hypothesis, which suggests otherwise.
Null and Alternative Hypotheses
Within hypothesis testing, the null hypothesis (often denoted as H0) assumes that any observed difference or effect is due to chance. This perspective is essential because it provides a baseline against which other observations can be compared. In contrast, the alternative hypothesis (denoted as or Ha) claims that there is indeed a relationship or difference present in the data.
The interplay between these two hypotheses forms the framework for statistical testing. One critical aspect of this framework is that it helps to maintain objectivity in data analysis. The null hypothesis stands as a defensive position that researchers must refute before accepting the alternative hypothesis. This approach has gained popularity due to its systematized nature, ensuring fewer biases in data interpretation. However, it does carry the downside of sometimes implying an absence of meaningful differences where they could exist.
Type and Type Errors
Errors arise in hypothesis testing, classified as Type I and Type II errors. A Type I error occurs when the null hypothesis is incorrectly rejected when it is true, meaning a false positive has been claimed ā quite like crying wolf when all is well. On the other hand, a Type II error happens when the null hypothesis fails to be rejected despite an actual difference being present ā akin to ignoring a real issue hiding in plain sight.
The balance between these errors is crucial. Striking the right balance often influences the outcome of studies significantly. Researchers must be cautious; while minimizing Type I errors can lead to safer conclusions, it often results in higher chances of Type II errors. Important decisions made based on misinterpretation of these hypotheses can lead to serious ramifications, especially in fields like healthcare and finance where stakes are high.
Understanding statistical inference, especially the subtleties of hypothesis testing and evaluation of errors, equips one to handle real-world data challenges more effectively. It creates a deeper understanding of the underlying processes and enhances the ability to make informed decisions.
Statistical Tests and Their Applications
In the realm of statistics, understanding the various tests and their applications is paramount. Statistical tests provide a framework that helps analysts determine the likelihood that a particular hypothesis is correct based on their data. They are the backbone of inferential statistics and essential for making informed decisions across various disciplines. In this section, we will explore two primary types of statistical tests: t-tests, ANOVA, and Chi-Square tests. Each of these tests can be pivotal in different scenarios, answering specific questions about data behavior and relationships.
t-tests and ANOVA
When it comes to comparing groups, two commonly used statistical tests are t-tests and ANOVA. The t-test is particularly useful when comparing the means of two groups. It examines whether the differences between the means of the groups are statistically significant or could have occurred by chance. This can apply in countless scenarios; think about medical research where a new drug is compared against a placebo.
In simple terms, a t-test can answer questions like: "Is the average recovery time shorter for patients taking the new medication compared to those taking the placebo?" If the p-value derived from the t-test is less than the significance level (typically set at 0.05), then the null hypothesis stating there is no difference can be rejected.
On the other hand, ANOVA (Analysis of Variance) comes into play when comparing the means of three or more groups. This test helps see if at least one of the group means is statistically different from the others. If you think beyond simple two-group comparisons, ANOVA is what you need. Imagine evaluating how different teaching methods impact student performance across three classes. Using ANOVA allows us to assess if any teaching style stands out regarding effectiveness.
A simple breakdown of when to use these tests:
- t-test: When comparing two groups, like patients on different medications.
- ANOVA: When comparing three or more groups, such as students in various teaching environments.
Both methods provide crucial insights that guide decisions in fields as varied as healthcare, business, and social sciences. Understanding the nuances of when to apply each test can significantly influence the outcomes of research studies and experiments.
Chi-Square Tests
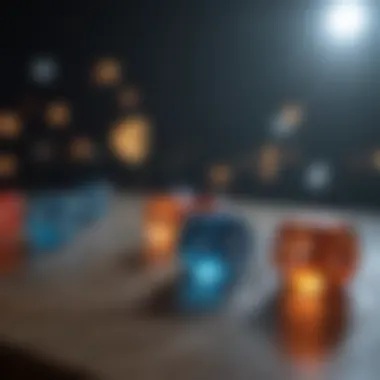
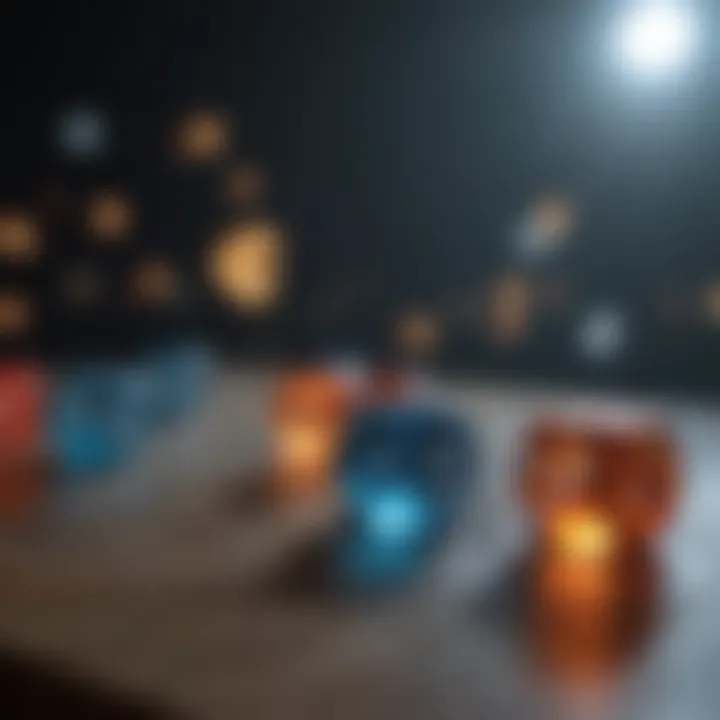
The Chi-Square test differs from t-tests and ANOVA as it is used primarily for categorical data. It examines the association between two categorical variables, essentially determining if the observed frequencies of the categories significantly deviate from what would be expected under the null hypothesis.
For example, consider a survey to see if thereās a relationship between gender (male or female) and preference for a product (like product A, B, or C). A Chi-Square test helps us analyze whether the distribution of preferences differs by gender. If there is a significant association, businesses can tailor marketing strategies to better reach specific demographics.
Hereās a quick look at the types of Chi-Square tests:
- Chi-Square Test of Independence: This checks if two categorical variables are independent of each other.
- Chi-Square Goodness-of-Fit Test: This assesses if the observed distribution of a single categorical variable fits an expected distribution.
In summary, statistical tests such as t-tests, ANOVA, and Chi-Square tests are invaluable tools in research and analysis. They allow statisticians and analysts to draw conclusions from data while controlling for the risk of error. Understanding how to apply the correct test ensures that decisions made from the statistical results are both valid and reliable. Like always, itās critical to comprehend the underlying assumptions and conditions of these tests before applying them, as improper use can lead to misleading results.
Understanding the right statistical test to apply can greatly enhance your analysis and prevent costly errors in interpretation.
Interpreting Statistical Results
Interpreting statistical results is a crucial aspect of understanding data analysis. It allows individuals to frame their insights into clear, actionable points. Misinterpretation can lead to flawed decisions, perceptions, and even policy formulations. This section will focus on two essential ideas: P-values and statistical significance and the common misinterpretations of statistics.
P-values and Statistical Significance
P-values have become a cornerstone in the evaluation of statistical results. Typically, a P-value indicates the probability that the observed data would occur by chance if the null hypothesis were true. In simplest terms, it helps in determining whether to accept or reject the null hypothesis. A smaller P-value (usually less than 0.05) signals that the observed trends in the data are statistically significant; thus, they are less likely to occur under the null hypothesis.
Hereās what one should consider when analyzing P-values:
- Thresholds of Significance: The common cutoff for statistical significance is 0.05, but this is not set in stone. In certain fields, more stringent criteria may be employed, say 0.01 or even 0.001. Using a consistent threshold across studies is essential for valid comparisons.
- Context of Research: Solely relying on P-values can lead to misleading conclusions. Understanding the research contextāsuch as sample size and effect sizeāis essential for sound interpretation.
- Anthropological Approach: Sociology and psychology fields often face challenges in adhering strictly to statistical significance. Practical relevance also matters, as a significant result does not always equate to substantial impact.
A P-value does not measure the probability that the null hypothesis is true or false. It merely indicates how compatible the data is with the null hypothesis.
Common Misinterpretations of Statistics
Statistics has its own language, and often this language is bent to fit narratives. Misinterpretations can arise in various scenarios, leading to skewed perceptions. Here are some common pitfalls:
- Confusion Between Correlation and Causation: One notable misstep is equating correlation with causation. Just because two variables move together doesnāt imply one causes the other. For instance, if ice cream sales increase concurrently with drowning incidents, it would be erroneous to conclude that eating ice cream leads to drowning. Itās vital to recognize lurking variables at play.
- Neglecting Sample Size: With small sample sizes, the results can be inherently unstable. The outcomes from just a handful of observations could be dramatically different with more data points, leading to overconfidence based on shaky ground.
- Ignoring the Margin of Error: Often, statistical representation fails to clarify margins of error, which can mislead public or academic perceptions. Reports of poll results may highlight averages but hide the variability present in sampling, leading to misguided interpretations of the data.
- Overemphasis on Significant Results: Itās easy to become enamored with statistically significant findings without considering other data nuances. Focusing solely on P-values may overlook meaningful insights embedded in the data.
In summary, interpreting statistical results is not just about crunching numbers. It requires a careful examination of the broader picture. Understanding P-values leads to informed inferences, while being wary of misinterpretations helps ground conclusions in reality. Both these components are indispensable for scholars, practitioners, and decision-makers navigating the complex world of data.
Statistical Software and Tools
In the vast realm of statistics, the tools we use can make a world of difference. Statistical software serves as the backbone for data analysis, ensuring accuracy and efficiency. The importance of this topic cannot be overstated, as the right tools can streamline complex computations, thereby freeing up time and resources while enhancing the overall quality of analyses.
Choosing a software tool isnāt just about picking the most popular option; itās about finding the right fit for your specific needs and expertise level. Whether you're an experienced statistician or just dipping your toes into the field, understanding the capabilities of various statistical software can make your data analysis journey smoother.
Foreword to Statistical Software
Statistical software has revolutionized data analysis, enabling users to handle large datasets, perform intricate calculations, and visualize results effectively. Each tool comes with its unique benefits and challenges, making it essential to comprehend their features before committing.
R and Python for Statistics
R and Python are two heavyweights in statistical computing. R, built specifically for statistics, offers an extensive variety of statistical techniques and a plethora of packages for various analyses. It's particularly known for its sophisticated plotting capabilities and its ability to handle complex data manipulation through packages such as ggplot2 and dplyr.
On the other hand, Python has risen to popularity due to its versatility and ease of learning. The integration of libraries like Pandas for data manipulation and NumPy for numerical calculations makes Python a powerful alternative.
Both R and Python have their strengths and weaknesses. R is adept at statistical modeling but can be less intuitive for those unfamiliar with coding. Python, while more approachable for general programming, may require additional libraries to match R's statistical breadth. Ultimately, choosing between them can depend on your project requirements and level of programming comfort.
SPSS and SAS
SPSS (Statistical Package for the Social Sciences) and SAS (Statistical Analysis System) are well-established tools often favored for their user-friendly interfaces. SPSS, designed primarily for social science researchers, simplifies statistical analysis, making it an appealing choice for those not interested in coding. It boasts a wide range of built-in functions for various types of analyses and offers an intuitive point-and-click interface.
SAS, a comprehensive tool used across industries, supports advanced analytics and business intelligence. Its scalability and extensive support for data manipulation make it valuable for handling large datasets. However, both SPSS and SAS come with licensing fees, which might deter some users.
Choosing the Right Tool for Analysis
When selecting a statistical software tool, consider the following factors:
- Purpose of Analysis: What types of analyses will you perform? Are they more descriptive or inferential?
- Familiarity: Are you comfortable with coding, or do you prefer a graphical interface?
- Budget: Can you afford the software? Look for open-source alternatives if budget is tight.
- Community Support: A large user community can be invaluable for troubleshooting and support.
Given the variety of tasks in statistics, it's a good idea to experiment with different tools to find what suits you best. Each tool has its unique ecosystem and strengths, so exploring options can help enhance your analytical skills.
Advanced Statistical Concepts
Understanding advanced statistical concepts is crucial for anyone keen on mastering the intricacies of data analysis. These concepts enable analysts and researchers to draw robust conclusions, make informed decisions, and develop predictive models based on complex datasets. Advanced statistics go beyond basic descriptive measures and delve deeper into understanding relationships and patterns within data, paving the way for innovation in various fields such as healthcare, economics, and social sciences.
Regression Analysis
Simple and Multiple Regression
Regression analysis serves as a backbone for statistical modeling, revealing correlations between variables. Simple regression involves two variables: one dependent and one independent. This is appreciably beneficial for grasping fundamental relationships, like predicting sales based on advertising spend; it's intuitive and gives immediate insights. On the flip side, multiple regression extends this by incorporating two or more independent variables to assess their simultaneous impact on a dependent variable. This offers a more nuanced understanding of complex relationships typical in real-world scenarios.
Its key characteristicāpredictive powerāmakes it a popular choice. Organizations may use multiple regression to forecast outcomes based on various influencing factors. For instance, when exploring real estate prices, variables such as square footage, location, and number of bedrooms are considered together.
However, one must tread carefully when utilizing these techniques. Overfitting is a potential drawback with multiple regression; it occurs when the model fits the training data too closely, making it less effective on new data. So, while multiple regression is an exceptionally powerful tool, it requires a strategic approach to avoid misleading results.
Assumptions of Regression
The assumptions underpinning regression analysis are pivotal to ensure reliable results. Each model rests on a set of conditions that must hold true for the analysis to be valid. For example, linearity, independence, and normality are fundamental. These assumptions help analysts determine whether the model appropriately represents the data without bias.
By explicitly addressing these assumptions, practitioners can assess the validity and reliability of their results. For example, suppose data deviates significantly from normal distribution; employing a non-linear model might yield superior insights. Thus, understanding and verifying these assumptions is a critical step in any regression analysis.
The unique feature of regression assumptions is their role in steering analysts towards effective model selection. By knowing what must be satisfied, those conducting the analysis can adjust techniques accordingly, seeking transformations or alternative analysis methods if necessary.
Non-parametric Methods
Moving beyond traditional statistical methods, non-parametric methods provide tools for analysis without requiring strict parameter assumptions. This is especially useful when dealing with small sample sizes or data that doesn't neatly fit into normal distributions.
Such techniques can illuminate relationships that parametric tests might overlook. For instance, in sociological studies, researchers might need to evaluate ordinal data or ranksānon-parametric tests like the Mann-Whitney U test become instrumental here. They allow for a rich exploration of data patterns without the constraints imposed by parametric tests.
Moreover, non-parametric methods are particularly advantageous in scenarios wherein sample sizes are unequal or when dealing with outliers. While they may lack the power of parametric tests under certain conditions, their flexibility makes them a valuable asset in the statistician's toolkit. Their adaptability signifies that they hold relevance across varied disciplines, enhancing the breadth of statistical investigation and fostering deeper data insights.
Ethics in Statistics
In the realm of statistics, ethics may not always steal the limelight, but it plays a pivotal role in shaping credible research and analysis. Just as a sailor relies on the stars to navigate the seas, statisticians depend on ethical guidelines to keep their work trustworthy and relevant. The integrity of data, the way it is presented, and the outcomes derived from it can have far-reaching implications, making a solid grasp of ethical considerations paramount.
When discussing ethics in statistics, it's vital to understand the consequences of data manipulation or misinterpretation. Noted instances where statistical data have been misrepresented have led to misguided conclusions in sectors such as health and policy-making. This doesn't just put a dent in the public's trust but can result in damage to lives and communities. Therefore, navigating the ethical landscape of statistics involves being forthright, objective, and respectful of the subject matter and its implications.
The importance of ethics in statistics is multi-faceted:
- Building Trust: Ethical practices foster a level of trust between statisticians, their audiences, and the subjects of their studies. When research is conducted ethically, stakeholders can believe in the outcomes reported.
- Preventing Misuse: Ethical considerations help prevent misuse of data. When ethical standards are upheld, the chances of skewing results or misapplying statistical tools decrease significantly.
- Societal Impact: Many statistical analyses can shape policies and public perception. Flawed or unethical data can lead to decisions that affect communities, businesses, and governance.
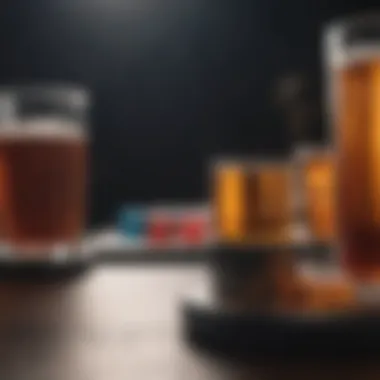
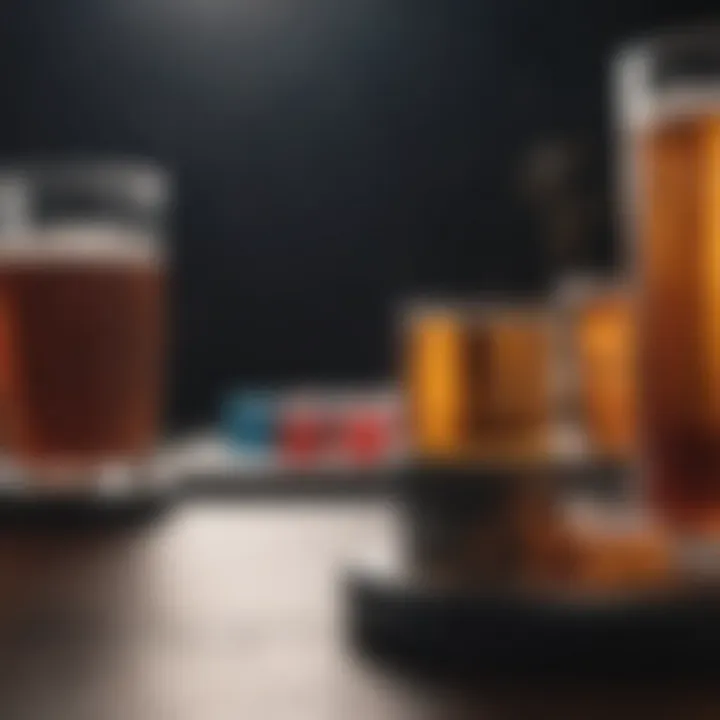
By upholding ethical standards, statisticians essentially ensure that their work serves the common good rather than a biased agenda. It is a professional commitment to accuracy and honesty that can not be overstated.
Ensuring Data Integrity
Ensuring data integrity is the bedrock of ethical statistical practice. Without it, whatever conclusions drawn from datasets become suspect, akin to building a house on sand. Data integrity refers to the accuracy, consistency, and reliability of data throughout its lifecycleāfrom collection through processing to analysis. Here are some key considerations for maintaining data integrity:
- Accurate Data Collection: Use reputable methods for gathering data, whether it's surveys, experiments, or observational studies. Data should be representative of the population under study.
- Transparent Methodologies: Always document your statistical methods. From selecting sampling techniques to the algorithms used in analysis, transparency allows others to replicate your findings.
- Regular Audits: Periodic checks and validation of the data help ensure that it remains accurate over time. This might involve comparing datasets against known standards or re-evaluating collection processes.
By maintaining vigilant oversight over data integrity, researchers not only protect their work but also uphold the integrity of the entire statistical discipline.
Ethical Considerations in Data Analysis
When it comes to data analysis, ethical considerations extend beyond data collection. Here, analysts must be wary of biases that can seep into their interpretations and reporting. It's easy to cherry-pick findings that fit a narrative, but professional integrity calls for a more holistic approach. Here are some key ethical aspects to consider:
- Objectivity in Analysis: Strive for impartiality. Even subtle biases in analysis can distort conclusions. Analysts should aim to consider all data equally and refrain from selectively interpreting findings to support a pre-existing belief or agenda.
- Reporting Results Honestly: Whether the results are favorable or not, accurately reporting what the data says is fundamental. Misleading representations, such as exaggerating statistical significance or failing to discuss limitations, erode trust.
- Respect for Confidentiality: If the data involves personal information, ensure that anonymity is preserved. Breaching confidentiality not only has ethical implications but can also lead to legal troubles.
In summary, ethics in statistics isn't a mere afterthought. Itās intertwined with the very practice of statistical analysis, influencing how data is collected, analyzed, and reported. As the saying goes, "With great power comes great responsibility." Statisticians wield significant power in interpreting data, and it is their responsibility to do so ethically, ensuring that their contributions are both valid and valuable.
Applications of Statistics in Various Fields
Statistics plays a pivotal role across multiple domains, providing essential insights that guide decisions. With its ability to distill complex data into understandable formats, one can see how it permeates business, healthcare, and social sciences. This section explores the importance, benefits, and various considerations regarding the application of statistics in these fields, ensuring a comprehensive understanding of its far-reaching impact.
Statistics in Business
In the realm of business, statistics is nothing short of a secret weapon. Companies leverage statistical analysis to make sense of market trends, consumer preferences, and operational efficiencies. Understanding customer behavior through sales data, for instance, allows firms to tailor products and marketing strategies effectively.
Businesses often employ tools like regression analysis to forecast future sales based on historical data. This predictive capability enables firms to allocate resources wisely, manage inventory better, and maximize profitability. Additionally, statistical methods are used in quality control processes. When checking production lines, businesses gather data to determine if their output meets quality standards.
Furthermore, statistical techniques, such as hypothesis testing, empower businesses to determine if changes in products or processes actually lead to improvements. In this interconnected landscape, a robust grasp of statistics can differentiate a thriving business from one struggling to keep pace.
Statistics in Healthcare
The healthcare field is another area where statistics shines brightly. From clinical trials to population health studies, the application of statistical methods ensures that medical decisions are backed by solid evidence. Statistical analysis aids in determining the effectiveness of new medications and medical devices through rigorous clinical trials. These trials often use randomized controlled methodologies to compare outcomes, allowing researchers to draw valid conclusions regarding efficacy and safety.
Moreover, statistics helps in tracking health trends and identifying health disparities within communities. By analyzing the incidence rates of certain diseases across different demographic groups, public health officials can allocate resources and prioritize interventions where they are most needed.
"Statistics drive evidence-based medicine, providing the framework by which healthcare professionals can evaluate treatment effectiveness."
Thus, in a world where patient outcomes matter enormously, statistics equips healthcare providers with the knowledge to make informed choices, ultimately enhancing patient care and outcomes.
Statistics in Social Sciences
In the social sciences, the role of statistics is fundamental in shaping research studies, informing policy decisions, and analyzing societal trends. Social scientists frequently rely on surveys to gather data about opinions and behaviors among various populations. By employing statistical methods, researchers can extrapolate their findings to broader societies, enhancing the understanding of social dynamics.
For instance, regression analysis can reveal correlations between educational attainment and income levels, leading to insights on social inequalities. Similarly, statistical models help researchers understand attitudes towards significant social issues, like climate change or healthcare access.
Given the complexity of human behavior, statistics offers a systematic approach to deciphering patterns, making it a crucial tool in the arsenal of scholars. While the subjective aspects of human behavior often resist easy quantitative measurement, statistical methods provide a framework that allows for meaningful analysis and interpretation.
As we can see, statistics permeates various fields, acting as a bedrock for decision-making processes, improving efficiency, and informing strategies. The relevance of statistics in business, healthcare, and social sciences underscores its value in navigating the complexities of the modern world.
Translating Statistical Knowledge into Practice
The world we inhabit today is profoundly shaped by data and insights derived from statistics. Translating statistical knowledge into practice becomes crucial for effectively using this knowledge to address real-life challenges. This section explores the importance of this translation, focusing on practical benefits and considerations that come with it.
Practicing statistical knowledge means more than just being able to perform calculations or interpret graphs. It's about applying statistical principles to draw meaningful conclusions from data and translating those conclusions into actionable strategies. As such, the relevance of this concept cannot be overstated. Without translating theory into practice, statistical insights can remain dormant, much like a book that gathers dust on a shelf.
Benefits of Translating Statistical Knowledge:
- Informed Decision-Making: By applying statistical methods, individuals and organizations can make decisions based on solid evidence rather than intuition alone.
- Increased Efficiency: Knowing how to apply statistical techniques can lead to more efficient processes, as data-driven decisions tend to eliminate guesswork.
- Enhanced Predictive Power: Statistical models can forecast trends, providing a competitive edge in various fields.
Considerations to Keep in Mind:
- Relevance to the Context: Always align statistical applications with context. The same method may yield different insights based on the setting.
- Ethical Implications: It's important to consider how statistics can be misused or misrepresented. Transparency in methodology fosters trust.
This translation process is essential in navigating today's data-rich landscape. The next subsections will illustrate real-world applications and how to bridge the gap between theoretical understanding and practical implementation.
Real-world Examples of Statistical Application
Grasping statistical concepts is one thing; applying them is a different ballgame. Numerous real-world examples demonstrate the practical implications of statistics in various domains. In healthcare, for instance, clinical trials utilize statistical methods to determine the effectiveness of new treatments. These methods help researchers assess whether the benefits of a medication outweigh its risks.
In the business sector, companies often employ statistics in market research to understand consumer behavior. By analyzing sales data and customer feedback, businesses can tailor their products or services to meet demand effectively. Similarly, think of economists who rely on statistical models to predict economic trends, influencing national policy decisions.
Furthermore, in environmental science, statistical analysis is employed to track climate change's impacts. Gathering and analyzing data on temperature, species populations, and other environmental indicators informs conservation efforts and policy initiatives.
"Statistics are like bikinis. What they reveal is suggestive, but what they conceal is vital." ā Aaron Levenstein.
These examples highlight how statistics serve as a powerful tool across disciplines, helping practitioners make informed decisions based on robust data analysis.
Bridging the Gap between Theory and Application
To truly capitalize on statistical knowledge, bridging the gap between theory and application is critical. This requires a thorough understanding of statistical concepts while also enhancing the ability to apply them successfully in practice.
Strategies for Bridging the Gap:
- Hands-on Experience: Engaging with real datasets through internships, research projects, or independent studies can solidify conceptual understandings.
- Collaboration with Experts: Working alongside experienced statisticians can provide insights into best practices and hone practical skills.
- Continual Learning: The field of statistics is ever-evolving, so stay updated with current trends, software, and methodologies.
By continually emphasizing the application of statistical knowledge, both novices and experts can find ways to enhance their analytical skills. Itās the difference between simply knowing the theory and being able to leverage that theory effectively to solve problems.
Statistical knowledge has the potential to transform perspectives and decision-making when effectively translated into practice. Understanding how to apply these ideas not only enriches personal knowledge but also becomes a critical aspect of professional growth in many fields.
Continuing Education in Statistics
Continuing education in statistics is crucial for anyone looking to sharpen their skills in this vast and ever-evolving field. Statistics isn't just a set of formulas or methods; it's a way of thinking and reasoning about the world around us. In our current data-driven society, the ability to interpret and analyze statistical data is more valuable than ever. As industries evolve and new technologies emerge, keeping your knowledge up-to-date is not just beneficial; itās imperative.
For those who are just dipping their toes into the world of statistics, ongoing education provides solid ground for building foundational knowledge. For seasoned professionals, it often means staying ahead of the curve. The landscape of statistics is changing rapidly. New methods, software, and applications pop up frequently, and those who neglect their education may soon find their skills outdated.
Benefits of Continuing Education in Statistics
- Staying Current: Regular learning helps you stay abreast of the latest techniques and innovations in statistical analysis.
- Enhanced Skills: Building on your existing knowledge means you can tackle more complex datasets and analyses effectively.
- Networking Opportunities: Engaging in courses, seminars, or webinars allows you to connect with industry professionals and expand your professional network.
- Career Advancement: Proactively seeking knowledge can propel you up the career ladder. Employers value those willing to invest time and effort into their own development.
- Confidence Boost: The more you learn, the more confident youāll feel in making decisions based on statistical data.
Many sectors such as finance, healthcare, and social sciences increasingly depend on advanced statistical methodologies. The more familiar you are with both the basics and the latest advancements, the more valuable you become in your respective field.
"Statistics is the language of science, and with the right education, anyone can become fluent."
Resources for Further Learning
Engaging with resources that cover various aspects of statistics can significantly enhance your understanding. Here are several options:
- Online Courses: Websites like Coursera and edX offer courses ranging from beginner to advanced levels, covering the fundamentals of statistics and its applications.
- Books: Titles like "The Elements of Statistical Learning" and "Naked Statistics" provide both depth and accessibility.
- Webinars and Workshops: Many professional organizations host sessions on specific topics in statisticsācheck out the American Statistical Association for upcoming events.
- Podcasts and Blogs: Listening to experts discuss statistical issues can provide insights into how statistics is applied in real-world scenarios.
- Documentation and Guides: Resources such as R Documentation or Python's NumPy Guide offer practical advice and examples for data analysis.
In summary, continuing education empowers individuals to grow within the field of statistics. By accessing the right resources, anyone can become adept at transforming data into actionable insights.